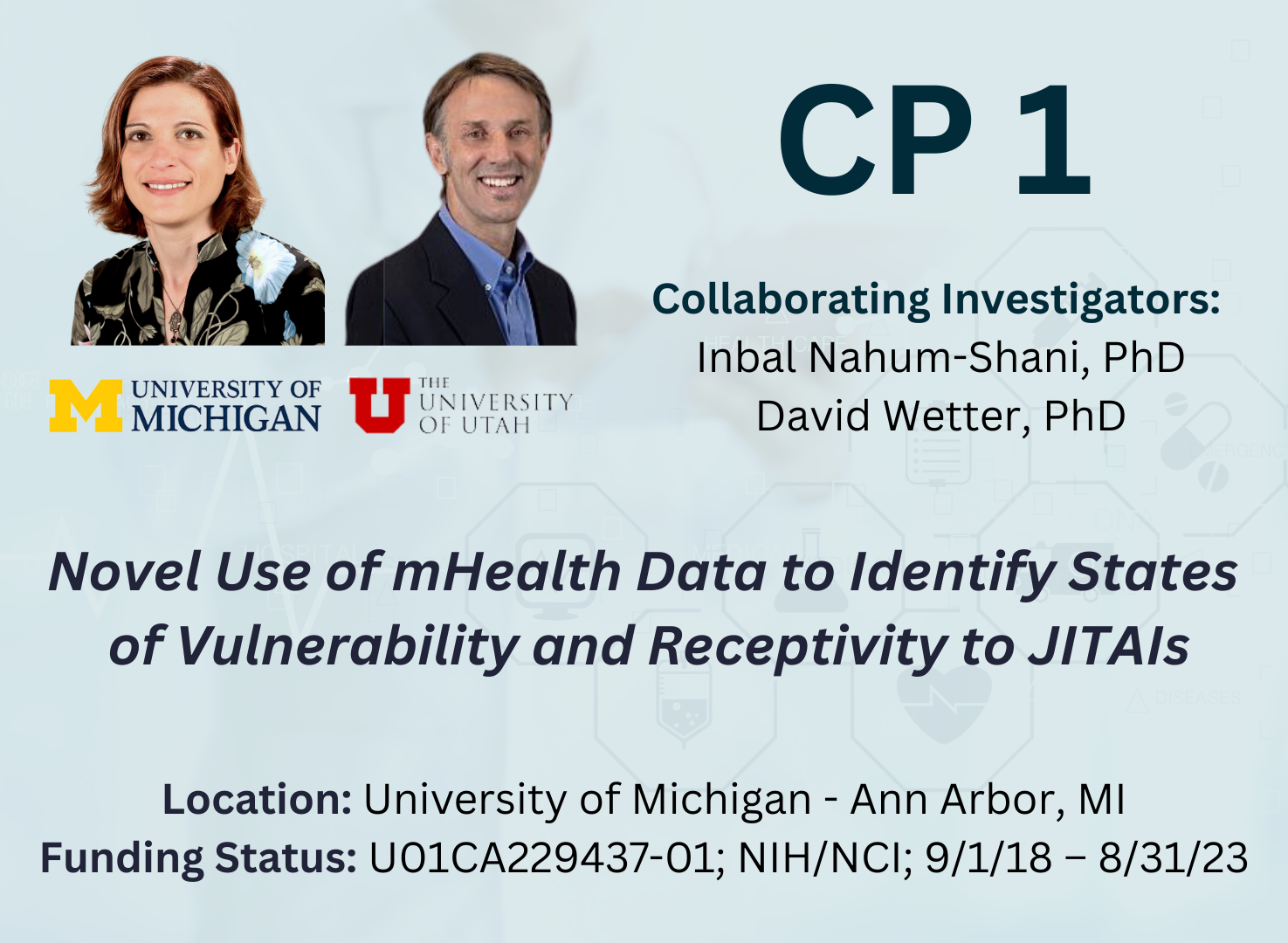
CP 1: Novel Use of mHealth Data to Identify States of Vulnerability and Receptivity to JITAIs
CP / Smoking Cessation / TR&D1 / TR&D2 / TR&D3
International Conference on Learning Representations (ICLR)
January 28, 2022
irregular sampling, uncertainty, imputation, interpolation, multivariate time series, missing data, variational autoencoder
In order to model and represent uncertainty in mHealth biomarkers to account for multifaceted uncertainty during momentary decision making in selecting, adapting, and delivering temporally-precise mHealth interventions. In this period, we extended our previous deep learning approach, Multi-Time Attention Networks, to enable improved representation of output uncertainty. Our new approach preserves the idea of learned temporal similarity functions and adds heteroskedastic output uncertainty. The new framework is referred to as the Heteroskedastic Variational Autoencoder and models real-valued multivariate data.
Irregularly sampled time series commonly occur in several domains where they present a significant challenge to standard deep learning models. In this paper, we propose a new deep learning framework for probabilistic interpolation of irregularly sampled time series that we call the Heteroscedastic Temporal Variational Autoencoder (HeTVAE). HeTVAE includes a novel input layer to encode information about input observation sparsity, a temporal VAE architecture to propagate uncertainty due to input sparsity, and a heteroscedastic output layer to enable variable uncertainty in output interpolations. Our results show that the proposed architecture is better able to reflect variable uncertainty through time due to sparse and irregular sampling than a range of baseline and traditional models, as well as recently proposed deep latent variable models that use homoscedastic output layers.
We present a new deep learning architecture for probabilistic interpolation of irregularly sampled time series.
IEEE/ACM international conference on Connected Health: Applications, Systems and Engineering Technologies (CHASE)
September 12, 2022
Bayesian inference, probabilistic programming, time series, missing data, Bayesian imputation, mobile health
We have developed a toolbox for the specification and estimation of mechanistic models in the dynamic bayesian network family. This toolbox focuses on making it easier to specify probabilistic dynamical models for time series data and to perform Bayesian inference and imputation in the specified model given incomplete data as input. The toolbox is referred to as BayesLDM. We have been working with members of CP3, CP4, and TR&D2 to develop offline data analysis and simulation models using this toolbox. We are also currently in discussions with members of CP4 to deploy the toolbox’s Bayesian imputation methods within a live controller optimization trial in the context of an adaptive walking intervention.
In this paper we present BayesLDM, a system for Bayesian longitudinal data modeling consisting of a high-level modeling language with specific features for modeling complex multivariate time series data coupled with a compiler that can produce optimized probabilistic program code for performing inference in the specified model. BayesLDM supports modeling of Bayesian network models with a specific focus on the efficient, declarative specification of dynamic Bayesian Networks (DBNs). The BayesLDM compiler combines a model specification with inspection of available data and outputs code for performing Bayesian inference for unknown model parameters while simultaneously handling missing data. These capabilities have the potential to significantly accelerate iterative modeling workflows in domains that involve the analysis of complex longitudinal data by abstracting away the process of producing computationally efficient probabilistic inference code. We describe the BayesLDM system components, evaluate the efficiency of representation and inference optimizations and provide an illustrative example of the application of the system to analyzing heterogeneous and partially observed mobile health data.
We present a a toolbox for the specification and estimation of mechanistic models in the dynamic bayesian network family.
Neural Information Processing Systems (NeurIPS), Track on Datasets and Benchmarks
September 16, 2022
missingness, imputation, mHealth, sensors, time-series, self-attention, pulsative, physiological, dataset
We developed a state-of-the-art attention-based deep learning transformer architecture that can learn to leverage the quasi-periodic signal structure to perform accurate imputation in the face of substantial amounts of missingness, such as the absence of multiple beats. We have validated that this novel transformer-based imputation method outperforms existing standard imputation baselines.
The promise of Mobile Health (mHealth) is the ability to use wearable sensors to monitor participant physiology at high frequencies during daily life to enable temporally-precise health interventions. However, a major challenge is frequent missing data. Despite a rich imputation literature, existing techniques are ineffective for the pulsative signals which comprise many mHealth applications, and a lack of available datasets has stymied progress. We address this gap with PulseImpute, the first large-scale pulsative signal imputation challenge which includes realistic mHealth missingness models, an extensive set of baselines, and clinically-relevant downstream tasks. Our baseline models include a novel transformer-based architecture designed to exploit the structure of pulsative signals. We hope that PulseImpute will enable the ML community to tackle this important and challenging task.
PulseImpute is the first mHealth pulsative signal imputation challenge which includes realistic missingness models, clinical downstream tasks, and an extensive set of baselines, including an augmented transformer that achieves SOTA performance.
Computer Vision – ECCV 2022 Workshops: Tel Aviv, Israel, Proceedings, Part VIII. Pages 78-93
February 12, 2023
transformer, uncertainty quantification, mixer, query-based obeject detection, deep ensembles
Recently, a new paradigm of query-based object detection has gained popularity. In this paper, we study the problem of quantifying the uncertainty in the predictions of these models that derive from model uncertainty. Such uncertainty quantification is vital for many high-stakes applications that need to avoid making overconfident errors. We focus on quantifying multiple aspects of detection uncertainty based on a deep ensembles representation. We perform extensive experiments on two representative models in this space: DETR and AdaMixer. We show that deep ensembles of these query-based detectors result in improved performance with respect to three types of uncertainty: location uncertainty, class uncertainty, and objectness uncertainty
This paper explores uncertainty in query-based object detection models, crucial for high-stakes applications to prevent overconfident errors. The authors concentrate on quantifying uncertainty in detection using deep ensembles, conducting experiments on DETR and AdaMixer models. They show that deep ensembles enhance performance in location, class, and objectness uncertainties.
Conference on Uncertainty in Artificial Intelligence (UAI 2023)
May 17, 2023
reinforcement learning, partial observability, context inference, adaptive interventions, empirical evaluation, mobile health
Just-in-Time Adaptive Interventions (JITAIs) are a class of personalized health interventions developed within the behavioral science community. JITAIs aim to provide the right type and amount of support by iteratively selecting a sequence of intervention options from a pre-defined set of components in response to each individual’s time varying state. In this work, we explore the application of reinforcement learning methods to the problem of learning intervention option selection policies. We study the effect of context inference error and partial observability on the ability to learn effective policies. Our results show that the propagation of uncertainty from context inferences is critical to improving intervention efficacy as context uncertainty increases, while policy gradient algorithms can provide remarkable robustness to partially observed behavioral state information.
This work focuses on JITAIs, personalized health interventions that dynamically select support components based on an individual’s changing state. The study applies reinforcement learning methods to learn policies for selecting intervention options, revealing that uncertainty from context inferences is crucial for enhancing intervention efficacy as context uncertainty increases.
Journal of Biomedical Informatics, Vol. 158
October 2024
System identification, Idiographic modeling, Dynamical systems modeling, Physical activity, Behavior change, Wearables
Digital behavior change interventions (DBCIs) are feasibly effective tools for addressing physical activity. However, in-depth understanding of participants’ long-term engagement with DBCIs remains sparse. Since the effectiveness of DBCIs to impact behavior change depends, in part, upon participant engagement, there is a need to better understand engagement as a dynamic process in response to an individual’s ever-changing biological, psychological, social, and environmental context. The year-long micro-randomized trial (MRT) HeartSteps II provides an unprecedented opportunity to investigate DBCI engagement among ethnically diverse participants. We combined data streams from wearable sensors (Fitbit Versa, i.e., walking behavior), the HeartSteps II app (i.e. page views), and ecological momentary assessments (EMAs, i.e. perceived intrinsic and extrinsic motivation) to build the idiographic models. A system identification approach and a fluid analogy model were used to conduct autoregressive with exogenous input (ARX) analyses that tested hypothesized relationships between these variables inspired by Self-Determination Theory (SDT) with DBCI engagement through time.
Data from 11 HeartSteps II participants was used to test aspects of the hypothesized SDT dynamic model. Across individuals, the number of daily notification prompts received by the participant was positively associated with increased app page views. The weekend/weekday indicator and perceived daily busyness were also found to be key predictors of the number of daily application page views. This novel approach has significant implications for both personalized and adaptive DBCIs by identifying factors that foster or undermine engagement in an individual’s respective context. Once identified, these factors can be tailored to promote engagement and support sustained behavior change over time.
This research explored long-term engagement with Digital Behavior Change Interventions (DBCIs) for physical activity using data from the year-long HeartSteps II trial. By combining data from wearables, app usage, and motivation assessments, the study developed models to understand engagement as a dynamic process. Key findings showed that daily notification prompts, whether it was a weekend or weekday, and perceived daily busyness were significant predictors of how often participants viewed the app pages. This novel approach has important implications for creating personalized and adaptive DBCIs that can better foster and sustain user engagement over time.
IFAC-PapersOnLine, Vol. 58, Iss. 15
October 2024
eHealth, Bayesian methods, Computational Social Sciences, Time series modelling
Digital behavior change interventions (DBCIs) such as “just-in-time” adaptive interventions (JITAIs) have demonstrated efficacy for increasing physical activity behavior. However, the effectiveness of these interventions is heavily dependent upon user engagement. Despite the inherent dynamic nature of engagement, as it varies over time based on an individual’s changing environment, context, and psychological state, the current understanding of engagement primarily comes from static snapshots of the behavior. The availability of intensive longitudinal data from JITAIs provides a unique opportunity to build and test dynamic models of behavior change from a process systems lens, relying on prediction-error methods from system identification. However, data missingness is a significant practical consideration in this process. Therefore, in this work we address missingness using a Bayesian imputation approach, which we evaluate using data from the HeartSteps II JITAI. Ultimately, the methods presented support the discovery of key factors that impact engagement behavior over time and can play an important role in the development of large-scale personalized interventions.
This publication focuses on using system identification and Bayesian imputation to create dynamic models of user engagement in mHealth digital behavior change interventions (DBCIs), such as “just-in-time” adaptive interventions (JITAIs). The goal is to move beyond static views of engagement to understand how it changes over time, addressing the practical issue of data missingness with a Bayesian approach. This research aims to identify key factors influencing engagement and support the development of personalized interventions, demonstrated using data from the HeartSteps II JITAI.
Martín Moreno
Advisor
Daniel E. Rivera
Committee Members
Arizona State University
August 2016
mHealth, behavioral interventions, Social Cognitive Theory (SCT), system identification, control engineering, physical inactivity, Hybrid Model Predictive Control (HMPC), Identification Test Monitoring (ITM), adaptive interventions, cyberphysical systems.
Behavioral health problems such as physical inactivity are among the main causes of mortality around the world. Mobile and wireless health (mHealth) interventions offer the opportunity for applying control engineering concepts in behavioral change settings. Social Cognitive Theory (SCT) is among the most influential theories of health behavior and has been used as the conceptual basis of many behavioral interventions. This dissertation examines adaptive behavioral interventions for physical inactivity problems based on SCT using system identification and control engineering principles. First, a dynamical model of SCT using fluid analogies is developed. The model is used throughout the dissertation to evaluate system identification approaches and to develop control strategies based on Hybrid Model Predictive Control (HMPC). An initial system identification informative experiment is designed to obtain basic insights about the system. Based on the informative experimental results, a second optimized experiment is developed as the solution of a formal constrained optimization problem. The concept of Identification Test Monitoring (ITM) is developed for determining experimental duration and adjustments to the input signals in real time. ITM relies on deterministic signals, such as multisines, and uncertainty regions resulting from frequency domain transfer function estimation that is performed during experimental execution. ITM is motivated by practical considerations in behavioral interventions; however, a generalized approach is presented for broad-based multivariable application settings such as process control. Stopping criteria for the experimental test utilizing ITM are developed using both open-loop and robust control considerations.
A closed-loop intensively adaptive intervention for physical activity is proposed relying on a controller formulation based on HMPC. The discrete and logical features of HMPC naturally address the categorical nature of the intervention components that include behavioral goals and reward points. The intervention incorporates online controller reconfiguration to manage the transition between the behavioral initiation and maintenance training stages. Simulation results are presented to illustrate the performance of the system using a model for a hypothetical participant under realistic conditions that include uncertainty. The contributions of this dissertation can ultimately impact novel applications of cyberphysical system in medical applications.
This dissertation presents a system identification and control engineering approach to optimize mobile health (mHealth) behavioral interventions, specifically addressing physical inactivity. It develops a dynamical model of Social Cognitive Theory (SCT) using fluid analogies, which is then used to evaluate system identification methods and develop control strategies based on Hybrid Model Predictive Control (HMPC). The work also introduces Identification Test Monitoring (ITM) procedures to determine the shortest necessary experimental duration while ensuring sufficient data for accurate model identification. Ultimately, these contributions aim to impact novel applications of cyberphysical systems in medical contexts.
ACM on Interactive, Mobile, Wearable, and Ubiquitous Technologies (IMWUT)
September 7, 2022
behavioral intervention, human-centered computing, risk prediction, smoking cessation, ubiquitous and mobile computing design and evaluation methods, wearable sensors
Passive detection of risk factors (that may influence unhealthy or adverse behaviors) via wearable and mobile sensors has created new opportunities to improve the effectiveness of behavioral interventions. A key goal is to find opportune moments for intervention by passively detecting rising risk of an imminent adverse behavior. But, it has been difficult due to substantial noise in the data collected by sensors in the natural environment and a lack of reliable label assignment of low- and high-risk states to the continuous stream of sensor data. In this paper, we propose an event-based encoding of sensor data to reduce the effect of noises and then present an approach to efficiently model the historical influence of recent and past sensor-derived contexts on the likelihood of an adverse behavior. Next, to circumvent the lack of any confirmed negative labels (i.e., time periods with no high-risk moment), and only a few positive labels (i.e., detected adverse behavior), we propose a new loss function. We use 1,012 days of sensor and self-report data collected from 92 participants in a smoking cessation field study to train deep learning models to produce a continuous risk estimate for the likelihood of an impending smoking lapse. The risk dynamics produced by the model show that risk peaks an average of 44 minutes before a lapse. Simulations on field study data show that using our model can create intervention opportunities for 85% of lapses with 5.5 interventions per day.
We present a model for identifying ideal moments for intervention by passively detecting risk of an imminent adverse behavior.
Neural Information Processing Systems (NeurIPS)
October 31, 2022
attention, continuous attention, kernel methods
One technical challenge in modeling missingness in biomarker streams is the need to develop flexible attention mechanisms that can learn to focus on the relevant aspects of an input signal. We have completed the development of a novel continuous-time attention model which is capable of learning multimodal densities, meaning that the attention density can be focused on multiple signal regions simultaneously. Classical solutions like Gaussian mixtures have dense support, with the result that all regions of a signal have some probability mass, making it difficult to focus the attention on key regions and ignore irrelevant ones. Our work introduces kernel deformed exponential families, a sparse class of multimodal attention densities.
We theoretically analysed the normalization, approximation, and numerical integration properties of this density class. We applied these densities in analyzing real-world time series data and showed that the densities often capture the most salient aspects of an input signal, and outperform baseline density models on a diverse set of tasks.
Attention mechanisms take an expectation of a data representation with respect to probability weights. Recently, (Martins et al. 2020, 2021) proposed continuous attention mechanisms, focusing on unimodal attention densities from the exponential and deformed exponential families: the latter has sparse support. (Farinhas et al 2021) extended this to to multimodality via Gaussian mixture attention densities. In this paper, we extend this to kernel exponential families (Canu and Smola 2006) and our new sparse counterpart, kernel deformed exponential families. Theoretically, we show new existence results for both kernel exponential and deformed exponential families, and that the deformed case has similar approximation capabilities to kernel exponential families. Lacking closed form expressions for the context vector, we use numerical integration: we show exponential convergence for both kernel exponential and deformed exponential families. Experiments show that kernel continuous attention often outperforms unimodal continuous attention, and the sparse variant tends to highlight peaks of time series.
We extend continuous attention from unimodal (deformed) exponential families and Gaussian mixture models to kernel exponential families and a new kernel deformed sparse counterpart.
Sy-Miin Chow, Inbal Nahum-Shani, Justin Baker, Donna Spruijt-Metz, Nicholas Allen, Randy Auerbach, Genevieve Dunton, Naomi Friedman, Stephen Intille, Predrag Klasnja, Benjamin Marlin, Matthew Nock, Scott Rauch, Misha Pavel, Scott Vrieze, David Wetter, Evan Kleiman, Timothy Brick, Heather Perry, Dana Wolff-Hughes
Translational Behavioral Medicine, Volume 13, Issue 1, January 2023, Pages 7–16
November 23, 2022
EMA, health behavior changes, ILHBN, location, sensor
The ILHBN is funded by the National Institutes of Health to collaboratively study the interactive dynamics of behavior, health, and the environment using Intensive Longitudinal Data (ILD) to (a) understand and intervene on behavior and health and (b) develop new analytic methods to innovate behavioral theories and interventions. The heterogenous study designs, populations, and measurement protocols adopted by the seven studies within the ILHBN created practical challenges, but also unprecedented opportunities to capitalize on data harmonization to provide comparable views of data from different studies, enhance the quality and utility of expensive and hard-won ILD, and amplify scientific yield. The purpose of this article is to provide a brief report of the challenges, opportunities, and solutions from some of the ILHBN’s cross-study data harmonization efforts. We review the process through which harmonization challenges and opportunities motivated the development of tools and collection of metadata within the ILHBN. A variety of strategies have been adopted within the ILHBN to facilitate harmonization of ecological momentary assessment, location, accelerometer, and participant engagement data while preserving theory-driven heterogeneity and data privacy considerations. Several tools have been developed by the ILHBN to resolve challenges in integrating ILD across multiple data streams and time scales both within and across studies. Harmonization of distinct longitudinal measures, measurement tools, and sampling rates across studies is challenging, but also opens up new opportunities to address cross-cutting scientific themes of interest.
The article shares insights, challenges, opportunities, and solutions from harmonizing intensive longitudinal data within the ILHBN, providing tools and recommendations for future data harmonization efforts.
Addictive Behaviors, Volume 136, p.107467
January 2023
just-in-time adaptive intervention, micro-randomized trial, mindfulness; smoking cessation; mHealth
Smoking cessation treatments that are easily accessible and deliver intervention content at vulnerable moments (e.g., high negative affect) have great potential to impact tobacco abstinence. The current study examined the feasibility and acceptability of a multi-component Just-In-Time Adaptive Intervention (JITAI) for smoking cessation. Daily smokers interested in quitting were consented to participate in a 6-week cessation study. Visit 1 occurred 4 days pre-quit, Visit 2 was on the quit day, Visit 3 occurred 3 days post-quit, Visit 4 was 10 days post-quit, and Visit 5 was 28 days post-quit. During the first 2 weeks (Visits 1-4), the JITAI delivered brief mindfulness/motivational strategies via smartphone in real-time based on negative affect or smoking behavior detected by wearable sensors. Participants also attended 5 in-person visits, where brief cessation counseling (Visits 1-4) and nicotine replacement therapy (Visits 2-5) were provided. Outcomes were feasibility and acceptability; biochemically-confirmed abstinence was also measured. Participants (N = 43) were 58.1 % female (AgeMean = 49.1, mean cigarettes per day = 15.4). Retention through follow-up was high (83.7 %). For participants with available data (n = 38), 24 (63 %) met the benchmark for sensor wearing, among whom 16 (67 %) completed at least 60 % of strategies. Perceived ease of wearing sensors (Mean = 5.1 out of 6) and treatment satisfaction (Mean = 3.6 out of 4) were high. Biochemically-confirmed abstinence was 34 % at Visit 4 and 21 % at Visit 5. Overall, the feasibility of this novel multi-component intervention for smoking cessation was mixed but acceptability was high. Future studies with improved technology will decrease participant burden and better detect key intervention moments.
The study assessed the feasibility and acceptability of a multi-component Just-In-Time Adaptive Intervention (JITAI) for smoking cessation, utilizing smartphone-delivered mindfulness/motivational strategies based on real-time negative affect or smoking behavior detected by wearable sensors. Participants showed high retention (83.7%) and reported high satisfaction with the intervention, but the feasibility was mixed.
ACM CHI 2024 – Under Review
Under Review
momentary stress, stressors, reflective visualizations, stressor logging, stress management, wearables
Our goal in Aim 3 is to understand the dynamic relationships between personalized drivers of momentary risk and disease progression to identify targets of temporally precise interventions. This year, we completed the MOODS study with 122 participants who wore a study-provided Fossil Sport smartwatch with our MOODS app, installed our MOODS app on their personal smartphones, and used both apps for 100 days. They rated their stress 3-4 times daily and described the stressor for events they rated as stressful. They received new visualizations of their data each week. We analyzed the impact of the study on self-reported stress ratings and the diversity in stressors reported by the participants.
Proceedings of the ACM on Interactive, Mobile, Wearable and Ubiquitous Technologies
mobile health, context, smoking cessation, intervention, GPS traces
March 2020
Context plays a key role in impulsive adverse behaviors such as fights, suicide attempts, binge-drinking, and smoking lapse. Several contexts dissuade such behaviors, but some may trigger adverse impulsive behaviors. We define these latter contexts as ‘opportunity’ contexts, as their passive detection from sensors can be used to deliver context-sensitive interventions. In this paper, we define the general concept of ‘opportunity’ contexts and apply it to the case of smoking cessation. We operationalize the smoking ‘opportunity’ context, using self-reported smoking allowance and cigarette availability. We show its clinical utility by establishing its association with smoking occurrences using Granger causality. Next, we mine several informative features from GPS traces, including the novel location context of smoking spots, to develop the SmokingOpp model for automatically detecting the smoking ‘opportunity’ context. Finally, we train and evaluate the SmokingOpp model using 15 million GPS points and 3,432 self-reports from 90 newly abstinent smokers in a smoking cessation study.
In this paper, we define the general concept of ‘opportunity’ contexts and apply it to the case of smoking cessation. We mine several informative features from GPS traces, including the novel location context of smoking spots, to develop the SmokingOpp model for automatically detecting the smoking ‘opportunity’ context.
Advances in Neural Information Processing Systems
irregular sampling, multivariate time series, missing data, discretization, interpolation, recurrence, attention
January 5, 2021
In this survey, we first describe several axes along which approaches to learning from irregularly sampled time series differ including what data representations they are based on, what modeling primitives they leverage to deal with the fundamental problem of irregular sampling, and what inference tasks they are designed to perform. We then survey the recent literature organized primarily along the axis of modeling primitives.
Contemporary Clinical Trials
engagement, Micro-randomized trial (MRT), mobile health (mHealth), self-regulatory strategies, smoking cessation
November 2021
Smoking is the leading preventable cause of death and disability in the U.S. Empirical evidence suggests that engaging in evidence-based self-regulatory strategies (e.g., behavioral substitution, mindful attention) can improve smokers’ ability to resist craving and build self-regulatory skills. However, poor engagement represents a major barrier to maximizing the impact of self-regulatory strategies. This paper describes the protocol for Mobile Assistance for Regulating Smoking (MARS) – a research study designed to inform the development of a mobile health (mHealth) intervention for promoting real-time, real-world engagement in evidence-based self-regulatory strategies. The study will employ a 10-day Micro-Randomized Trial (MRT) enrolling 112 smokers attempting to quit. Utilizing a mobile smoking cessation app, the MRT will randomize each individual multiple times per day to either: (a) no intervention prompt; (b) a prompt recommending brief (low effort) cognitive and/or behavioral self-regulatory strategies; or (c) a prompt recommending more effortful cognitive or mindfulness-based strategies. Prompts will be delivered via push notifications from the MARS mobile app. The goal is to investigate whether, what type of, and under what conditions prompting the individual to engage in self-regulatory strategies increases engagement. The results will build the empirical foundation necessary to develop a mHealth intervention that effectively utilizes intensive longitudinal self-report and sensor-based assessments of emotions, context and other factors to engage an individual in the type of self-regulatory activity that would be most beneficial given their real-time, real-world circumstances. This type of mHealth intervention holds enormous potential to expand the reach and impact of smoking cessation treatments.
This paper describes the protocol for Mobile Assistance for Regulating Smoking (MARS) – a research study designed to inform the development of a mobile health (mHealth) intervention for promoting real-time, real-world engagement in evidence-based self-regulatory strategies.
Yun C Zhang, Shibo Zhang, Miao Liu, Elyse Daly, Samuel Battalio, Santosh Kumar, Bonnie Spring, James M Rehg, Nabil Alshurafa
Proceedings of the ACM on Interactive, Mobile, Wearable and Ubiquitous Technologies
September 4, 2024
Accelerometry, Automatic Synchronization, Temporal Drift, Time Synchronization, Video, Wearable Camera, Wearable Sensor. Additional relevant keywords from the index terms include Human-centered computing, Ubiquitous and mobile computing, Ubiquitous and mobile computing design and evaluation methods, and Ubiquitous and mobile computing systems and tools.
The development and validation of computational models to detect daily human behaviors (e.g., eating, smoking, brushing) using wearable devices requires labeled data collected from the natural field environment, with tight time synchronization of the micro-behaviors (e.g., start/end times of hand-to-mouth gestures during a smoking puff or an eating gesture) and the associated labels. Video data is increasingly being used for such label collection. Unfortunately, wearable devices and video cameras with independent (and drifting) clocks make tight time synchronization challenging. To address this issue, we present the Window Induced Shift Estimation method for Synchronization (SyncWISE) approach. We demonstrate the feasibility and effectiveness of our method by synchronizing the timestamps of a wearable camera and wearable accelerometer from 163 videos representing 45.2 hours of data from 21 …
SyncWISE is a novel method for accurately synchronizing data from wearable cameras and accelerometers, crucial for developing computational models to detect daily human behaviors from natural field environments. It addresses the challenge of independent and drifting clocks between devices, demonstrating significant improvement over existing methods even with high data loss, achieving 90% synchronization accuracy within a 700-millisecond tolerance.
Proceedings of the 2024 CHI Conference on Human Factors in Computing Systems
May 11, 2024
Stress-tracking, Stressor-logging, Visualizations, Behavioral Changes, Stress Intervention, Wearable Sensors, Field studies, Empirical studies in HCI, Self-awareness, Personal informatics.
Commercial wearables from Fitbit, Garmin, and Whoop have recently introduced real-time notifications based on detecting changes in physiological responses indicating potential stress. In this paper, we investigate how these new capabilities can be leveraged to improve stress management. We developed a smartwatch app, a smartphone app, and a cloud service, and conducted a 100-day field study with 122 participants who received prompts triggered by physiological responses several times a day. They were asked whether they were stressed, and if so, to log the most likely stressor. Each week, participants received new visualizations of their data to self-reflect on patterns and trends. Participants reported better awareness of their stressors, and self-initiating fourteen kinds of behavioral changes to reduce stress in their daily lives. Repeated self-reports over 14 weeks showed reductions in both stress intensity …
This 100-day field study, named MOODS, investigated how wearable-triggered stressor logging and weekly self-reflective data visualizations can improve stress management. Participants used a smartwatch to detect physiological events and a smartphone app to log perceived stress and specific stressors when prompted. The study found that this approach increased participants’ awareness of their stressors, motivated them to initiate 14 types of behavioral changes, and resulted in statistically significant reductions in both stress intensity (11%) and stress frequency (9.5%). The findings suggest that enabling easy stressor logging and providing insightful visualizations could be a powerful component in future stress management apps.
Bipolar Disorders Conference, Vol 25, pg. 34
June 1, 2023
depressive symptoms, daily stressors, young adults, affective responsivity, mental health, stress appraisal, sex differences, anger, shame, negative affect, positive affect, subclinical depression, psychosocial stress, stress generation theory, daily diary study.
Greater negative affective responsivity (NA-R) to daily stress is associated with increased risk of cardiovascular disease (CVD); however, the underlying mechanisms remain unclear. Given that heightened blood pressure (BP) reactivity to stress is also purported to increase future CVD risk, we hypothesized that increased NA-R to daily stress would be positively related to greater BP and heart rate (HR) reactivity to acute stress. Because major depressive disorder (MDD) is characterized by stress system dysfunction, we further hypothesized that the slope of this relation would be steeper in adults with MDD compared to healthy non-depressed adults (HA). Participants (n = 38 HA/n = 30 MDD; 18–30 yrs) completed the Daily Inventory of Stressful Events (DISE) interview every day for 8 consecutive days to quantify objective (and subjective appraisal characteristics (of naturally-occurring daily stressors. On DISE Day 8, beat-to-beat mean arterial pressure (MAP; finger photoplethysmography) and HR (single-lead ECG) were measured during the cold pressor test. In all participants (n = 68), greater NA-R was positively related to the magnitude of the CPT-induced increase in MAP (β = 13.69, SE = 7.54, p = 0.07) and HR (β = 18.27, SE = 9.00, p = 0.04). Compared to HA, NA-R was greater in adults with MDD (0.26 ± 0.10 vs. 0.36 ± 0.17 a.u.; p < 0.01). Further, the relation between NA-R and BP and, separately, HR reactivity to the CPT were steeper in adults with MDD (MAP: β = 23.25, SE = 8.97, p = 0.02; HR: β = 24.77, SE = 9.33, p = 0.01) compared to HA (both p > 0.05). These data suggest that greater negative affective responsivity is associated with greater acute cardiovascular stress reactivity and that this relation is sensitized in adults with MDD.
This study reveals that young adults with more severe depressive symptoms — even those without a formal diagnosis — report daily stressors more pervasively and experience a greater worsening of negative affect in response to them. Specifically, higher symptom severity is linked to more frequent stressor exposure, increased anger and shame post-stressor, and amplified negative (and modulated positive) affective responsivity to daily stressors, which may elevate their risk for future chronic diseases.
Autonomic Neuroscience, Vol 259, pg. 259
June 1, 2025
Major Depressive Disorder, sympathetic nervous system, muscle sympathetic nerve activity, emotional stress, negative images, International Affective Picture System, cardiovascular disease risk, young women, microneurography, depressive symptom severity, blood pressure.
Young women, who suffer from major depressive disorder (MDD) at twice the rate as young men, are particularly vulnerable to cardiovascular events triggered by emotional stress, an association that may be partially explained by excessive sympathetic activation. However, no studies have directly measured sympathetic activity during acute emotional stress in young women with MDD. We hypothesized that the muscle sympathetic nerve activity (MSNA) response to acute emotional stress would be greater in young women with MDD (18–30 yrs) compared to healthy non-depressed young women. MSNA (peroneal microneurography) and beat-to-beat blood pressure (BP; finger photoplethysmography) were measured at rest and during acute emotional stress evoked by viewing negative images selected from the International Affective Picture System in 17 healthy young women and in 30 young women with MDD of …
Young women with mild-to-moderate Major Depressive Disorder (MDD) did not show exaggerated sympathetic nerve activity (MSNA) or blood pressure (BP) responses to passive emotional stress (viewing negative images) compared to healthy women. However, the study found that in women with MDD, greater current depressive symptom severity was positively related to a larger MSNA response to emotional stress, suggesting that more severe symptoms might amplify sympathetic activation and potentially increase cardiovascular disease risk.
Emerging Adulthood, Vol 13, S1, pg. 214
February 2025
daily diary, negative affect, positive affect, daily stress, emotion, young adulthood, sex differences.
Despite mounting evidence that young adults experience greater exposure and affective responsivity to daily stressors than middle-aged and older adults, few studies have examined potential sex differences in these daily stress processes in young adults. We tested the hypotheses that young women would experience (1) a greater percentage of days with at least one daily stressor event and (2) exaggerated negative and positive affective responsivity to daily stressors compared to young men. Young adults (n = 215) completed a daily web-based interview for eight consecutive days to assess multiple dynamic aspects of daily stress processes. Women experienced a greater frequency of daily stressor days. Further, the magnitude of the difference in both negative and positive affect between stressor-free and stressor days were greater in young women compared to young men. Greater exposure and amplified …
This study reveals that young women not only encounter daily stressors more frequently but also experience a significantly amplified emotional response—both negative and positive—to these stressors compared to young men. This heightened affective responsivity and increased exposure to daily stressors may contribute to a greater vulnerability to adverse psychological outcomes in young women.
Journal of Applied Physiology
May 5, 2025
Potential keyword tags that can be associated with this publication include: stress, negative affect, daily stressors, norepinephrine excretion, middle-aged adults, biological markers, affective responsivity.
Despite mounting evidence that greater affective responsivity to naturally occurring daily stressors is associated with increased risk of cardiovascular diseases (CVDs), few studies have examined dysregulation of the sympathetic nervous system as a potential mechanism. We hypothesized that greater affective responsivity to daily stressful events would be related to increased urinary catecholamine excretion. Daily stress processes (8-day daily diary) were assessed in 715 middle-aged adults (56 ± 11 yr; 57% female) from the Midlife in the United States Study. Urinary norepinephrine and epinephrine concentrations were also measured (24 h; normalized to creatinine). Multilevel modeling was used to calculate negative and positive affective responsivity (i.e., the slope of the within-person differences in negative and positive affect on stressor days compared with stressor-free days). Analyses controlled for relevant…
This publication, titled “Greater negative affective responsivity to daily stressors is positively related to urinary norepinephrine excretion in middle-aged adults”, explores the relationship where a more pronounced negative emotional reaction to everyday stressors is linked to higher levels of norepinephrine being excreted in the urine of middle-aged adults.
Medicine & Science in Sports & Exercise, Vol 55, S9, pg. 409
September 1, 2023
Cardiovascular Health, Blood Pressure Dipping, Physical Activity, Stress Reactivity, Hormonal Cycles, Vascular Function, Chronic Ankle Instability, Muscle Physiology, Lactate Response, Para-Athletes.
This collection of research abstracts investigates various aspects of human physiology, including the impact of habitual physical activity on cardiovascular health, such as nocturnal blood pressure dipping and stress reactivity. It presents findings on the influence of hormonal cycles on vascular function, examines muscle performance in chronic ankle instability, and explores unique physiological responses in para-athletes. The studies offer insights ranging from the non-effect of sodium loading on BP dipping in young adults to the positive association between habitual physical activity and BP dipping, and the lack of moderation by physical activity on stress-induced heart rate responses.
Heteroscedastic Temporal Variational Autoencoder For Irregularly Sampled Time Series
https://github.com/reml-lab/hetvae
13 forks.
32 stars.
4 open issues.
Recent commits:
International Conference on Learning Representations (ICLR)
January 28, 2022
Jupyter Notebook
Python
BayesLDM
https://github.com/reml-lab/BayesLDM
1 forks.
0 stars.
0 open issues.
Recent commits:
IEEE/ACM international conference on Connected Health: Applications, Systems and Engineering Technologies (CHASE)
September 12, 2022
Code for PulseImpute Challenge
https://github.com/rehg-lab/pulseimpute
1 forks.
28 stars.
0 open issues.
Recent commits:
Neural Information Processing Systems (NeurIPS)
September 16, 2022
Code for ECCV 2022 UnCV Workshop paper
https://github.com/colinski/uq-query-object-detectors
0 forks.
0 stars.
0 open issues.
Recent commits:
Computer Vision – ECCV 2022 Workshops: Tel Aviv, Israel, Proceedings, Part VIII. Pages 78-93
February 12, 2023
Markdown Documentation
RL for JITAI optimization using simulated environments.
https://github.com/reml-lab/rl_jitai_simulation
0 forks.
1 stars.
0 open issues.
Recent commits:
Conference on Uncertainty in Artificial Intelligence (UAI 2023)
May 17, 2023
Predicting Smoking Lapse Risk from Mobile Sensor Datastreams
https://github.com/aungkonazim/mrisk
0 forks.
0 stars.
0 open issues.
Recent commits:
ACM on Interactive, Mobile, Wearable, and Ubiquitous Technologies (IMWUT)
September 7, 2022
Jupyter Notebook
Python
Code for Kernel Multimodal Continuous Attention
https://github.com/onenoc/kernel-continuous-attention
1 forks.
3 stars.
0 open issues.
Recent commits:
Neural Information Processing Systems (NeurIPS)
October 31, 2022
Jupyter Notebook
Python
International Conference on Learning Representations (ICLR)
January 28, 2022
The novel PulseImpute dataset is the first large-scale dataset containing complex imputation tasks for pulsative biophysical signals. State-of-the-art imputation methods from the time series literature are shown to exhibit poor performance on PulseImpute, demonstrating that the missingness patterns emerging in mHealth applications represent a unique and important class of imputation problems. By releasing this dataset and a new state-of-the-art baseline algorithm, we hope to spur the ML community to begin addressing these challenging problems.
Neural Information Processing Systems (NeurIPS)
November 28, 2022
The past decade has seen tremendous advances in the ability to compute a diverse array of mobile sensor-based biomarkers in order to passively estimate health states, activities, and associated contexts (e.g. physical activity, sleep, smoking, mood, craving, stress, and geospatial context). Researchers are now engaged in the conduct of both observational and interventional field studies of increasing complexity and length that leverage mHealth sensor and biomarker technologies combined with the collection of measures of disease progression and other outcomes.
As a result of the expansion of the set of available mHealth biomarkers and the push toward long-term, real-world deployment of mHealth technologies, a new set of critical gaps has emerged that were previously obscured by the focus of the field on smaller-scale proof-of-concept studies and the investigation of single biomarkers in isolation.
First, the issue of missing sensor and biomarker data in mHealth field studies has quickly become a critical problem that directly and significantly impacts many of our CPs. Issues including intermittent wireless dropouts, wearables and smartphones running out of battery power, participants forgetting to carry or wear devices, and participants exercising privacy controls can all contribute to complex patterns of missing data that significantly complicate data analysis and limit the effectiveness of sensor-informed mHealth interventions.
Second, with increasing interest in the use of reinforcement learning methods to provide online adaptation of interventions for every individual, there is an urgent need for high-quality, compact and interpretable feature representations that can enable more effective learning under strict budgets on the number of interactions with patients.
Finally, as in other areas that are leveraging machine learning methods to drive scientific discovery and support decision making, mHealth needs methods that can be used to derive high-level knowledge and support causal hypothesis generation based on complex, non-linear models fit to biomarker time series data.
Assistant Professor
Applied Scientist
Principal Algorithms Engineer
Senior Research Scientist
Applied Scientist II
Applied Scientist
Machine Learning Scientist
Deputy Center Director, TR&D1 Lead
Lead PI, Center Director, TR&D1, TR&D2, TR&D3
Co-Investigator, TR&D1, TR&D2
Doctoral Student
Doctoral Student
Doctoral Student
TR&D1’s technologies are making a significant impact by advancing the fundamental understanding of health and behavior by supporting the analysis of complex, longitudinal, mHealth data.