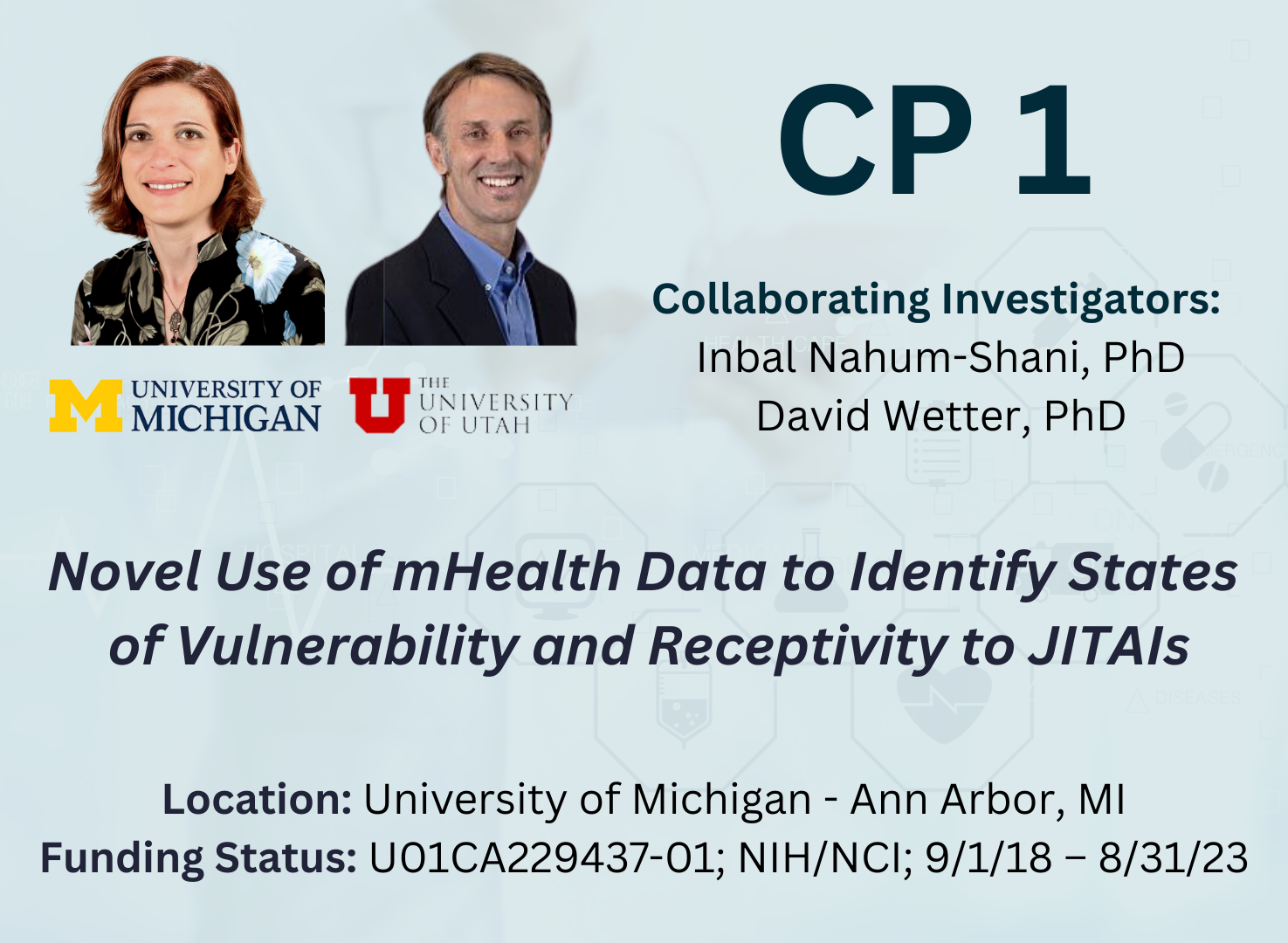
CP 1: Novel Use of mHealth Data to Identify States of Vulnerability and Receptivity to JITAIs
CP / Smoking Cessation / TR&D1 / TR&D2 / TR&D3
Algorithms in Decision Support Systems
July 22, 2022
reinforcement learning, online learning, mobile health, algorithm design, algorithm evaluation
Online RL faces challenges like real-time stability and handling complex, unpredictable environments; to address these issues, the PCS framework originally used in supervised learning is extended to guide the design of RL algorithms for such settings, including guidelines for creating simulation environments, as exemplified in the development of an RL algorithm for the mobile health study Oralytics aimed at enhancing tooth-brushing behaviors through personalized intervention messages.
Advances in Neural Information Processing Systems
December 2021
contextual bandit algorithms, confidence intervals, adaptively collected data, causal inference
We develop theory justifying the use of M-estimators—which includes estimators based on empirical risk minimization as well as maximum likelihood—on data collected with adaptive algorithms, including (contextual) bandit algorithms.
Conference on Innovative Applications of Artificial Intelligence (IAAI 2023)
February 7, 2023
reinforcement learning, online learning, mobile health, algorithm design, algorithm evaluation
Dental disease is one of the most common chronic diseases despite being largely preventable. However, professional advice on optimal oral hygiene practices is often forgotten or abandoned by patients. Therefore patients may benefit from timely and personalized encouragement to engage in oral self-care behaviors. In this paper, we develop an online reinforcement learning (RL) algorithm for use in optimizing the delivery of mobile-based prompts to encourage oral hygiene behaviors. One of the main challenges in developing such an algorithm is ensuring that the algorithm considers the impact of the current action on the effectiveness of future actions (i.e., delayed effects), especially when the algorithm has been made simple in order to run stably and autonomously in a constrained, real-world setting (i.e., highly noisy, sparse data). We address this challenge by designing a quality reward which maximizes the desired health outcome (i.e., high-quality brushing) while minimizing user burden. We also highlight a procedure for optimizing the hyperparameters of the reward by building a simulation environment test bed and evaluating candidates using the test bed. The RL algorithm discussed in this paper will be deployed in Oralytics, an oral self-care app that provides behavioral strategies to boost patient engagement in oral hygiene practices.
In this paper, we develop an online reinforcement learning (RL) algorithm for use in optimizing the delivery of mobile-based prompts to encourage oral hygiene behaviors.
April 19, 2023
Online reinforcement learning and other adaptive sampling algorithms are increasingly used in digital intervention experiments to optimize treatment delivery for users over time. In this work, we focus on longitudinal user data collected by a large class of adaptive sampling algorithms that are designed to optimize treatment decisions online using accruing data from multiple users. Combining or “pooling” data across users allows adaptive sampling algorithms to potentially learn faster. However, by pooling, these algorithms induce dependence between the sampled user data trajectories; we show that this can cause standard variance estimators for i.i.d. data to underestimate the true variance of common estimators on this data type. We develop novel methods to perform a variety of statistical analyses on such adaptively sampled data via Z-estimation. Specifically, we introduce the adaptive sandwich variance estimator, a corrected sandwich estimator that leads to consistent variance estimates under adaptive sampling. Additionally, to prove our results we develop novel theoretical tools for empirical processes on non-i.i.d., adaptively sampled longitudinal data which may be of independent interest. This work is motivated by our efforts in designing experiments in which online reinforcement learning algorithms optimize treatment decisions, yet statistical inference is essential for conducting analyses after experiments conclude.
arXiv:2306.10983
June 27, 2023
effect-invariant mechanisms, policy generalization, machine learning
arXiv:2307.13916
October 31, 2023
contextual bandits, predicted context, online learning, machine learning
We consider the contextual bandit problem where at each time, the agent only has access to a noisy version of the context and the error variance (or an estimator of this variance). This setting is motivated by a wide range of applications where the true context for decision-making is unobserved, and only a prediction of the context by a potentially complex machine learning algorithm is available. When the context error is non-vanishing, classical bandit algorithms fail to achieve sublinear regret. We propose the first online algorithm in this setting with sublinear regret guarantees under mild conditions. The key idea is to extend the measurement error model in classical statistics to the online decision-making setting, which is nontrivial due to the policy being dependent on the noisy context observations. We further demonstrate the benefits of the proposed approach in simulation environments based on synthetic and real digital intervention datasets.
We propose the first online algorithm in this setting with sublinear regret guarantees under mild conditions.
arXiv:2305.18511
May 29, 2023
machine learning, optimization and control, contextual bandits, information reveal
Contextual bandit algorithms are commonly used in digital health to recommend personalized treatments. However, to ensure the effectiveness of the treatments, patients are often requested to take actions that have no immediate benefit to them, which we refer to as pro-treatment actions. In practice, clinicians have a limited budget to encourage patients to take these actions and collect additional information. We introduce a novel optimization and learning algorithm to address this problem. This algorithm effectively combines the strengths of two algorithmic approaches in a seamless manner, including 1) an online primal-dual algorithm for deciding the optimal timing to reach out to patients, and 2) a contextual bandit learning algorithm to deliver personalized treatment to the patient. We prove that this algorithm admits a sub-linear regret bound. We illustrate the usefulness of this algorithm on both synthetic and real-world data.
We present an innovative optimization and learning algorithm to tackle the challenge clinicians face with constrained budgets, aiming to incentivize patients to take actions and gather additional information.
Proceedings of Machine Learning Research 149:1–50
contextual bandits, meta-algorithms, mobile health
August 2021
Contextual bandits often provide simple and effective personalization in decision making problems, making them popular tools to deliver personalized interventions in mobile health as well as other health applications. However, when bandits are deployed in the context of a scientific study — e.g. a clinical trial to test if a mobile health intervention is effective — the aim is not only to personalize for an individual, but also to determine, with sufficient statistical power, whether or not the system’s intervention is effective. It is essential to assess the effectiveness of the intervention before broader deployment for better resource allocation. The two objectives are often deployed under different model assumptions, making it hard to determine how achieving the personalization and statistical power affect each other. In this work, we develop general meta-algorithms to modify existing algorithms such that sufficient power is guaranteed while still improving each user’s well-being. We also demonstrate that our meta-algorithms are robust to various model mis-specifications possibly appearing in statistical studies, thus providing a valuable tool to study designers.
In this work, we develop general meta-algorithms to modify existing algorithms such that sufficient power is guaranteed while still improving each user’s well-being. We also demonstrate that our meta-algorithms are robust to various model mis-specifications possibly appearing in statistical studies, thus providing a valuable tool to study designers.
NAM Perspectives
mhealth interventions, mobile health
August 2021
Mobile Health (mHealth) technologies are now commonly used to deliver interventions in a self-service and personalized manner, reducing the demands on providers and lifting limitations on the locations in which care can be delivered.
Advances in Neural Information Processing Systems
bached bandits, ordinary least squares estimator
January 8, 2021
Statistical Science: a review journal of the Institute of Mathematical Statistics
causal inference, endogenous covariates, linear mixed model, micro-randomized trial
October 2020
Mobile health is a rapidly developing field in which behavioral treatments are delivered to individuals via wearables or smartphones to facilitate health-related behavior change. Micro-randomized trials (MRT) are an experimental design for developing mobile health interventions. In an MRT the treatments are randomized numerous times for each individual over course of the trial. Along with assessing treatment effects, behavioral scientists aim to understand between-person heterogeneity in the treatment effect. A natural approach is the familiar linear mixed model. However, directly applying linear mixed models is problematic because potential moderators of the treatment effect are frequently endogenous-that is, may depend on prior treatment. We discuss model interpretation and biases that arise in the absence of additional assumptions when endogenous covariates are included in a linear mixed model. In particular, when there are endogenous covariates, the coefficients no longer have the customary marginal interpretation. However, these coefficients still have a conditional-on-the-random-effect interpretation. We provide an additional assumption that, if true, allows scientists to use standard software to fit linear mixed model with endogenous covariates, and person-specific predictions of effects can be provided. As an illustration, we assess the effect of activity suggestion in the HeartSteps MRT and analyze the between-person treatment effect heterogeneity.
We discuss model interpretation and biases that arise in the absence of additional assumptions when endogenous covariates are included in a linear mixed model. In particular, when there are endogenous covariates, the coefficients no longer have the customary marginal interpretation.
Journal of the American Statistical Association
sequential decision making, policy evaluation, markov decision process, reinforcement learning
2021
In this paper, we provide an approach for conducting inference about the performance of one or more such policies using historical data collected under a possibly different policy.
Current Addiction Reports
addiction, just-in-time adaptive intervention, micro-randomized trial, mobile health
September 2020
Addiction is a serious and prevalent problem across the globe. An important challenge facing intervention science is how to support addiction treatment and recovery while mitigating the associated cost and stigma. A promising solution is the use of mobile health (mHealth) just-in-time adaptive interventions (JITAIs), in which intervention options are delivered in situ via a mobile device when individuals are most in need.
The present review describes the use of mHealth JITAIs to support addiction treatment and recovery, and provides guidance on when and how the micro-randomized trial (MRT) can be used to optimize a JITAI. We describe the design of five mHealth JITAIs in addiction and three MRT studies, and discuss challenges and future directions.
This review aims to provide guidance for constructing effective JITAIs to support addiction treatment and recovery.
The Annals of Statistics
December 21, 2022
average reward, doubly robust estimator, Markov Decision Process, policy optimization
We consider the batch (off-line) policy learning problem in the infinite horizon Markov decision process. Motivated by mobile health applications, we focus on learning a policy that maximizes the long-term average reward. We propose a doubly robust estimator for the average reward and show that it achieves semiparametric efficiency. Further, we develop an optimization algorithm to compute the optimal policy in a parameterized stochastic policy class. The performance of the estimated policy is measured by the difference between the optimal average reward in the policy class and the average reward of the estimated policy and we establish a finite-sample regret guarantee. The performance of the method is illustrated by simulation studies and an analysis of a mobile health study promoting physical activity.
We consider batch policy learning in an infinite horizon Markov Decision Process, focusing on optimizing a policy for long-term average reward in the context of mobile health applications.
IEEE International Conference on Digital Health (ICDH)
July 10, 2022
learning systems, optimized production technology, behavioral sciences, electronic healthcare, decision trees
To promote healthy behaviors, many mobile health applications provide message-based interventions, such as tips, motivational messages, or suggestions for healthy activities. Ideally, the intervention policies should be carefully designed so that users obtain the benefits without being overwhelmed by overly frequent messages. As part of the HeartSteps physical-activity intervention, users receive messages intended to disrupt sedentary behavior. HeartSteps uses an algorithm to uniformly spread out the daily message budget over time, but does not attempt to maximize treatment effects. This limitation motivates constructing a policy to optimize the message delivery decisions for more effective treatments. Moreover, the learned policy needs to be interpretable to enable behavioral scientists to examine it and to inform future theorizing. We address this problem by learning an effective and interpretable policy that reduces sedentary behavior. We propose Optimal Policy Trees + (OPT+), an innovative batch off-policy learning method, that combines a personalized threshold learning and an extension of Optimal Policy Trees under a budget-constrained setting. We implement and test the method using data collected in HeartSteps V2N3. Computational results demonstrate a significant reduction in sedentary behavior with a lower delivery budget. OPT + produces a highly interpretable and stable output decision tree thus enabling theoretical insights to guide future research.
Online RL faces challenges like real-time stability and handling complex, unpredictable environments; to address these issues, the PCS framework originally used in supervised learning is extended to guide the design of RL algorithms for such settings, including guidelines for creating simulation environments, as exemplified in the development of an RL algorithm for the mobile health study Oralytics aimed at enhancing tooth-brushing behaviors through personalized intervention messages.
NIH National Library of Medicine: National Center for Biotechnology Information – ClinicalTrials.gov, Identifier: NCT05624489
Submission Under Review
engagement strategies, dental disease, health behavior change, oral self-care behaviors
The study will involve a 10-week Micro-Randomized Trial (MRT) to inform the delivery of prompts (via mobile app push notifications) designed to facilitate adherence to an ideal tooth brushing protocol (2x2x4; 2 sessions daily, 2 minutes per session, all 4 quadrants).
arXiv:2304.05365v6
August 7, 2023
reinforcement learning, personalization, resampling, exploratory data analysis, mobile health
There is a growing interest in using reinforcement learning (RL) to personalize sequences of treatments in digital health to support users in adopting healthier behaviors. Such sequential decision-making problems involve decisions about when to treat and how to treat based on the user’s context (e.g., prior activity level, location, etc.). Online RL is a promising data-driven approach for this problem as it learns based on each user’s historical responses and uses that knowledge to personalize these decisions. However, to decide whether the RL algorithm should be included in an “optimized” intervention for real-world deployment, we must assess the data evidence indicating that the RL algorithm is actually personalizing the treatments to its users. Due to the stochasticity in the RL algorithm, one may get a false impression that it is learning in certain states and using this learning to provide specific treatments. We use a working definition of personalization and introduce a resampling-based methodology for investigating whether the personalization exhibited by the RL algorithm is an artifact of the RL algorithm stochasticity. We illustrate our methodology with a case study by analyzing the data from a physical activity clinical trial called HeartSteps, which included the use of an online RL algorithm. We demonstrate how our approach enhances data-driven truth-in-advertising of algorithm personalization both across all users as well as within specific users in the study.
We use a working definition of personalization and introduce a resampling-based methodology for investigating whether the personalization exhibited by the RL algorithm is an artifact of the RL algorithm stochasticity.
JMIR Formative Research
December 11, 2023
engagement, oral health, mobile health intervention, racial and ethnic minority group, message development
Background: The prevention of oral health diseases is a key public health issue and a major challenge for racial and ethnic minority groups, who often face barriers in accessing dental care. Daily toothbrushing is an important self-care behavior necessary for sustaining good oral health, yet engagement in regular brushing remains a challenge. Identifying strategies to promote engagement in regular oral self-care behaviors among populations at risk of poor oral health is critical.
Objective: The formative research described here focused on creating messages for a digital oral self-care intervention targeting a racially and ethnically diverse population. Theoretically grounded strategies (reciprocity, reciprocity-by-proxy, and curiosity) were used to promote engagement in 3 aspects: oral self-care behaviors, an oral care smartphone app, and digital messages. A web-based participatory co-design approach was used to develop messages that are resource efficient, appealing, and novel; this approach involved dental experts, individuals from the general population, and individuals from the target population—dental patients from predominantly low-income racial and ethnic minority groups. Given that many individuals from racially and ethnically diverse populations face anonymity and confidentiality concerns when participating in research, we used an approach to message development that aimed to mitigate these concerns.
Methods: Messages were initially developed with feedback from dental experts and Amazon Mechanical Turk workers. Dental patients were then recruited for 2 facilitator-mediated group webinar sessions held over Zoom (Zoom Video Communications; session 1: n=13; session 2: n=7), in which they provided both quantitative ratings and qualitative feedback on the messages. Participants interacted with the facilitator through Zoom polls and a chat window that was anonymous to other participants. Participants did not directly interact with each other, and the facilitator mediated sessions by verbally asking for message feedback and sharing key suggestions with the group for additional feedback. This approach plausibly enhanced participant anonymity and confidentiality during the sessions.
Results: Participants rated messages highly in terms of liking (overall rating: mean 2.63, SD 0.58; reciprocity: mean 2.65, SD 0.52; reciprocity-by-proxy: mean 2.58, SD 0.53; curiosity involving interactive oral health questions and answers: mean 2.45, SD 0.69; curiosity involving tailored brushing feedback: mean 2.77, SD 0.48) on a scale ranging from 1 (do not like it) to 3 (like it). Qualitative feedback indicated that the participants preferred messages that were straightforward, enthusiastic, conversational, relatable, and authentic.
Conclusions: This formative research has the potential to guide the design of messages for future digital health behavioral interventions targeting individuals from diverse racial and ethnic populations. Insights emphasize the importance of identifying key stimuli and tasks that require engagement, gathering multiple perspectives during message development, and using new approaches for collecting both quantitative and qualitative data while mitigating anonymity and confidentiality concerns.
The formative research described here focused on creating messages for a digital oral self-care intervention targeting a racially and ethnically diverse population. Theoretically grounded strategies (reciprocity, reciprocity-by-proxy, and curiosity) were used to promote engagement in 3 aspects: oral self-care behaviors, an oral care smartphone app, and digital messages.
To appear in Volume 20 of the Annual Review of Clinical Psychology, 2023
2023
engagement, oral health, mobile health intervention, racial and ethnic minority group, message development
JMIR Research Protocols
ecological momentary assessment, adolescents, young adults, oncology, cancer, self-management, mobile health (mHealth)
October 22, 2021
Background: Adolescents and young adults (AYAs) with cancer demonstrate suboptimal oral chemotherapy adherence, increasing their risk of cancer relapse. It is unclear how everyday time-varying contextual factors (eg, mood) affect their adherence, stalling the development of personalized mobile health (mHealth) interventions. Poor engagement is also a challenge across mHealth trials; an effective adherence intervention must be engaging to promote uptake.
Objective: This protocol aims to determine the temporal associations between daily contextual factors and 6-mercaptopurine (6-MP) adherence and explore the proximal impact of various engagement strategies on ecological momentary assessment survey completion.
Methods: At the Children s Hospital of Philadelphia, AYAs with acute lymphoblastic leukemia or lymphoma who are prescribed prolonged maintenance chemotherapy that includes daily oral 6-MP are eligible, along with their matched caregivers. Participants will use an ecological momentary assessment app called ADAPTS (Adherence Assessments and Personalized Timely Support) a version of an open-source app that was modified for AYAs with cancer through a user-centered process and complete surveys in bursts over 6 months. Theory-informed engagement strategies will be microrandomized to estimate the causal effects on proximal survey completion.
Results: With funding from the National Cancer Institute and institutional review board approval, of the proposed 30 AYA-caregiver dyads, 60% (18/30) have been enrolled; of the 18 enrolled, 15 (83%) have completed the study so far.
Conclusions: This protocol represents an important first step toward prescreening tailoring variables and engagement components for a just-in-Time adaptive intervention designed to promote both 6-MP adherence and mHealth engagement.
This protocol represents an important first step toward prescreening tailoring variables and engagement components for a just-in-Time adaptive intervention designed to promote both 6-MP adherence and mHealth engagement.
Machine Learning, Volume 110, Pages 2685–2727
Thompson-Sampling, mobile health, reinforcement learning
September 2021
In mobile health (mHealth) smart devices deliver behavioral treatments repeatedly over time to a user with the goal of helping the user adopt and maintain healthy behaviors. Reinforcement learning appears ideal for learning how to optimally make these sequential treatment decisions. However, significant challenges must be overcome before reinforcement learning can be effectively deployed in a mobile healthcare setting. In this work we are concerned with the following challenges: 1) individuals who are in the same context can exhibit differential response to treatments 2) only a limited amount of data is available for learning on any one individual, and 3) non-stationary responses to treatment. To address these challenges we generalize Thompson-Sampling bandit algorithms to develop IntelligentPooling. IntelligentPooling learns personalized treatment policies thus addressing challenge one. To address the second challenge, IntelligentPooling updates each user’s degree of personalization while making use of available data on other users to speed up learning. Lastly, IntelligentPooling allows responsivity to vary as a function of a user’s time since beginning treatment, thus addressing challenge three. We show that IntelligentPooling achieves an average of 26% lower regret than state-of-the-art. We demonstrate the promise of this approach and its ability to learn from even a small group of users in a live clinical trial.
To address the significant challenges that must be overcome before reinforcement learning can be deployed in a mobile healthcare setting, we develop IntelligentPooling by generalizing Thompson-Sampling bandit algorithms.
Current Opinion in Systems Biology
adolescent, young adult, chemotherapy adherance, micro-randomized trial
June 2020
Long-term engagement with mobile health (mHealth) apps can provide critical data for improving empirical models for real-time health behaviors. To learn how to improve and maintain mHealth engagement, micro-randomized trials (MRTs) can be used to optimize different engagement strategies. In MRTs, participants are sequentially randomized, often hundreds or thousands of times, to different engagement strategies or treatments. The data gathered are then used to decide which treatment is optimal in which context. In this paper, we discuss an example MRT for youth with cancer, where we randomize different engagement strategies to improve self-reports on factors related to medication adherence. MRTs, moreover, can go beyond improving engagement, and we reference other MRTs to address substance abuse, sedentary behavior, and so on.
In this paper, we discuss an example MRT for youth with cancer, where we randomize different engagement strategies to improve self-reports on factors related to medication adherence.
Psychiatry: Interpersonal and Biological Processes
July 18, 2022
suicide, self-injury, just-in-time adaptive interventions
The suicide rate (currently 14 per 100,000) has barely changed in the United States over the past 100 years. There is a need for new ways of preventing suicide. Further, research has revealed that suicidal thoughts and behaviors and the factors that drive them are dynamic, heterogeneous, and interactive. Most existing interventions for suicidal thoughts and behaviors are infrequent, not accessible when most needed, and not systematically tailored to the person using their own data (e.g., from their own smartphone). Advances in technology offer an opportunity to develop new interventions that may better match the dynamic, heterogeneous, and interactive nature of suicidal thoughts and behaviors. Just-In-Time Adaptive Interventions (JITAIs), which use smartphones and wearables, are designed to provide the right type of support at the right time by adapting to changes in internal states and external contexts, offering a promising pathway toward more effective suicide prevention. In this review, we highlight the potential of JITAIs for suicide prevention, challenges ahead (e.g., measurement, ethics), and possible solutions to these challenges.
In this review, we highlight the potential of JITAIs for suicide prevention, challenges ahead (e.g., measurement, ethics), and possible solutions to these challenges.
American Psychologist
March 17, 2022
engagement, digital interventions, affect, motivation, attention
The notion of “engagement,” which plays an important role in various domains of psychology, is gaining increased currency as a concept that is critical to the success of digital interventions. However, engagement remains an ill-defined construct, with different fields generating their own domain-specific definitions. Moreover, given that digital interactions in real-world settings are characterized by multiple demands and choice alternatives competing for an individual’s effort and attention, they involve fast and often impulsive decision making. Prior research seeking to uncover the mechanisms underlying engagement has nonetheless focused mainly on psychological factors and social influences and neglected to account for the role of neural mechanisms that shape individual choices. This paper aims to integrate theories and empirical evidence across multiple domains to define engagement and discuss opportunities and challenges to promoting effective engagement in digital interventions. We also propose the AIM-ACT framework, which is based on a neurophysiological account of engagement, to shed new light on how in-the-moment engagement unfolds in response to a digital stimulus. Building on this framework, we provide recommendations for designing strategies to promote engagement in digital interventions and highlight directions for future research.
This paper focuses on defining and understanding engagement in digital interventions by combining various theories and evidence from different domains. It introduces the AIM-ACT framework, which explains how engagement happens in response to digital stimuli based on neurophysiological principles and offers suggestions for designing effective engagement strategies in digital interventions.
Psychological Methods
January 13, 2022
December 2021
engagement, mobile health (mHealth), Micro-Randomized Trial (MRT), reciprocity, reinforcement
Contemporary Clinical Trials
engagement, Micro-randomized trial (MRT), mobile health (mHealth), self-regulatory strategies, smoking cessation
November 2021
Smoking is the leading preventable cause of death and disability in the U.S. Empirical evidence suggests that engaging in evidence-based self-regulatory strategies (e.g., behavioral substitution, mindful attention) can improve smokers’ ability to resist craving and build self-regulatory skills. However, poor engagement represents a major barrier to maximizing the impact of self-regulatory strategies. This paper describes the protocol for Mobile Assistance for Regulating Smoking (MARS) – a research study designed to inform the development of a mobile health (mHealth) intervention for promoting real-time, real-world engagement in evidence-based self-regulatory strategies. The study will employ a 10-day Micro-Randomized Trial (MRT) enrolling 112 smokers attempting to quit. Utilizing a mobile smoking cessation app, the MRT will randomize each individual multiple times per day to either: (a) no intervention prompt; (b) a prompt recommending brief (low effort) cognitive and/or behavioral self-regulatory strategies; or (c) a prompt recommending more effortful cognitive or mindfulness-based strategies. Prompts will be delivered via push notifications from the MARS mobile app. The goal is to investigate whether, what type of, and under what conditions prompting the individual to engage in self-regulatory strategies increases engagement. The results will build the empirical foundation necessary to develop a mHealth intervention that effectively utilizes intensive longitudinal self-report and sensor-based assessments of emotions, context and other factors to engage an individual in the type of self-regulatory activity that would be most beneficial given their real-time, real-world circumstances. This type of mHealth intervention holds enormous potential to expand the reach and impact of smoking cessation treatments.
This paper describes the protocol for Mobile Assistance for Regulating Smoking (MARS) – a research study designed to inform the development of a mobile health (mHealth) intervention for promoting real-time, real-world engagement in evidence-based self-regulatory strategies.
Conference on Uncertainty in Artificial Intelligence (UAI 2023)
May 17, 2023
reinforcement learning, partial observability, context inference, adaptive interventions, empirical evaluation, mobile health
Just-in-Time Adaptive Interventions (JITAIs) are a class of personalized health interventions developed within the behavioral science community. JITAIs aim to provide the right type and amount of support by iteratively selecting a sequence of intervention options from a pre-defined set of components in response to each individual’s time varying state. In this work, we explore the application of reinforcement learning methods to the problem of learning intervention option selection policies. We study the effect of context inference error and partial observability on the ability to learn effective policies. Our results show that the propagation of uncertainty from context inferences is critical to improving intervention efficacy as context uncertainty increases, while policy gradient algorithms can provide remarkable robustness to partially observed behavioral state information.
This work focuses on JITAIs, personalized health interventions that dynamically select support components based on an individual’s changing state. The study applies reinforcement learning methods to learn policies for selecting intervention options, revealing that uncertainty from context inferences is crucial for enhancing intervention efficacy as context uncertainty increases.
arXiv:2308.07843
November 3, 2023
dyadic reinforcement learning, online learning, mobile health, algorithm design
Mobile health aims to enhance health outcomes by delivering interventions to individuals as they go about their daily life. The involvement of care partners and social support networks often proves crucial in helping individuals managing burdensome medical conditions. This presents opportunities in mobile health to design interventions that target the dyadic relationship — the relationship between a target person and their care partner — with the aim of enhancing social support. In this paper, we develop dyadic RL, an online reinforcement learning algorithm designed to personalize intervention delivery based on contextual factors and past responses of a target person and their care partner. Here, multiple sets of interventions impact the dyad across multiple time intervals. The developed dyadic RL is Bayesian and hierarchical. We formally introduce the problem setup, develop dyadic RL and establish a regret bound. We demonstrate dyadic RL’s empirical performance through simulation studies on both toy scenarios and on a realistic test bed constructed from data collected in a mobile health study.
In this paper, we develop dyadic RL, an online reinforcement learning algorithm designed to personalize intervention delivery based on contextual factors and past responses of a target person and their care partner.
Digital Therapeutics for Mental Health and Addiction (pp.77-87)
just-in-time adaptive interventions, mental health, addiction
January 2023
Advances in mobile and sensing technologies offer many opportunities for delivering just-in-time adaptive interventions (JITAIs)—interventions that use dynamically changing information about the individual’s internal state and context to recommend whether and how to deliver interventions in real-time, in daily life. States of vulnerability to an adverse outcome and states of receptivity to a just-in-time intervention play a critical role in the formulation of effective JITAIs. However, these states are defined, operationalized, and studied in various ways across different fields and research projects. This chapter is intended to (a) clarify the definition and operationalization of vulnerability to adverse outcomes and receptivity to just-in-time interventions; and (b) provide greater specificity in formulating scientific questions about these states. This greater precision has the potential to aid researchers in selecting the most suitable study design for answering questions about states of vulnerability and receptivity to inform JITAIs.
States of vulnerability to an adverse outcome and states of receptivity to a just-in-time intervention play a critical role in the formulation of effective JITAIs. This chapter is intended to clarify the definition and operationalization of vulnerability to adverse outcomes and receptivity to just-in-time interventions; and provide greater specificity in formulating scientific questions about these states.
Biometrika
binary outcome, causal excursion effect, causal inference, longitudinal data, micro-randomized trials, mobile health, relative risk, semiparametric efficiency theory
September 2021
We develop an estimator that can be used as the basis of a primary aim analysis under more plausible assumptions. Simulation studies are conducted to compare the estimators. We illustrate the developed methods using data from the MRT, BariFit. In BariFit, the goal is to support weight maintenance for individuals who received bariatric surgery.
Contemporary Clinical Trials
digital intervention, just-in-time adaptive intervention, micro-randomized trial, optimization, smoking, stress, mHealth
August 8, 2021
Background: Relapse to smoking is commonly triggered by stress, but behavioral interventions have shown only modest efficacy in preventing stress-related relapse. Continuous digital sensing to detect states of smoking risk and intervention receptivity may make it feasible to increase treatment efficacy by adapting intervention timing.
Objective: Aims are to investigate whether the delivery of a prompt to perform stress management behavior, as compared to no prompt, reduces the likelihood of (a) being stressed and (b) smoking in the subsequent two hours, and (c) whether current stress moderates these effects.
Study Design: A micro-randomized trial will be implemented with 75 adult smokers who wear Autosense chest and wrist sensors and use the mCerebrum suite of smartphone apps to report and respond to ecological momentary assessment (EMA) questions about smoking and mood for 4 days before and 10 days after a quit attempt and to access a set of stress-management apps. Sensor data will be processed on the smartphone in real time using the cStress algorithm to classify minutes as probably stressed or probably not stressed. Stressed and non-stressed minutes will be micro-randomized to deliver either a prompt to perform a stress management exercise via one of the apps or no prompt (2.5-3 stress management prompts will be delivered daily). Sensor and self-report assessments of stress and smoking will be analyzed to optimize decision rules for a just-in-time adaptive intervention (JITAI) to prevent smoking relapse.
Significance: Sense2Stop will be the first digital trial using wearable sensors and micro-randomization to optimize a just-in-time adaptive stress management intervention for smoking relapse prevention.
Sense2Stop will be the first digital trial using wearable sensors and micro-randomization to optimize a just-in-time adaptive stress management intervention for smoking relapse prevention.
Code for "Designing Reinforcement Learning Algorithms for Digital Interventions: Pre-implementation Guidelines" Paper
https://github.com/StatisticalReinforcementLearningLab/pcs-for-rl
0 forks.
2 stars.
0 open issues.
Recent commits:
Algorithms in Decision Support Systems
July 22, 2022
Python
Code to reproduce results for "Statistical Inference with M-Estimators on Adaptively Collected Data"
https://github.com/kellywzhang/adaptively_weighted_Mestimation
1 forks.
2 stars.
0 open issues.
Recent commits:
Advances in Neural Information Processing Systems
December 2021
Python
Shell
The material in this repository is a supplement to the manuscript titled `The Mobile-Assistance for Regulating Smoking (MARS) Micro-Randomized Trial Design Protocol' (Nahum-Shani, et al., 2021), submitted for consideration to the Journal of Contemporary Clinical Trials. The material include code and documentation for the power calculation for the Primary Aim and Secondary Aim of the trial.
https://github.com/jamieyap/power-calc-mars-mrt
1 forks.
0 stars.
0 open issues.
Recent commits:
Contemporary Clinical Trials
November 2021
R
RL for JITAI optimization using simulated environments.
https://github.com/reml-lab/rl_jitai_simulation
0 forks.
0 stars.
0 open issues.
Recent commits:
arXiv:2308.07843
November 3, 2023
Python
R
Shell
https://github.com/mDOT-Center/pJITAI
2 forks.
1 stars.
44 open issues.
Recent commits:
The pJITAI toolbox being developed in TR&D2 software and code repository. The repository serves as a centralized hub for storing, managing, and tracking the evolution of project code, scripts, and software tools. This facilitates seamless collaboration among project members, streamlining the process of code integration, debugging, and enhancement. The repository’s branching and versioning capabilities enable the team to work concurrently on different aspects of the project without compromising code integrity. It ensures that changes are tracked, reviewed, and merged in a controlled manner, bolstering the project’s overall reliability.
Mobile health (mHealth) interventions have typically used hand-crafted decision rules that map from biomarkers of an individual’s state to the selection of interventions. Recently, reinforcement learning (RL) has emerged as a promising approach for online optimization of decision rules. Continuous, passive detection of the individual’s state using mHealth biomarkers enables dynamic deployment of decision rules at the right moment, i.e., as and when events of interest are detected from sensors. RL-based optimization methods that leverage this new capability created by sensor-based biomarkers, can enable the development and optimization of temporally-precise mHealth interventions, overcoming the significant limitations of static, one-size-fits-all decision rules. Such next generation interventions have the potential to lead to greater treatment efficacy and improved long-term engagement.
However, there exist several critical challenges to the realization of effective, real-world RL-based interventions including the need to learn efficiently based on limited interactions with an individual while accounting for longer-term effects of intervention decisions, (i.e., to avoid habituation and ensure continued engagement), and accommodating multiple intervention components operating at different time scales and targeting different outcomes. As a result, the use of RL in mHealth interventions has mostly been limited to very few studies using basic RL methods.
To address these critical challenges, TR&D2 builds on more precise biomarkers of context, including TR&D1 risk and engagement scores, to develop, evaluate, and disseminate robust and data efficient RL methods and tools. These methods continually personalize the selection, adaptation and delivery timing decision rules for core intervention components so as to maximize long-term therapeutic efficacy and engagement for every individual.
Assistant Professor
Applied Scientist II
Senior Research Scientist
Applied Scientist
Presentations by Susan Murphy
Presentations by Raaz Dwivedi (postdoctoral researcher)
Presentation by Kyra Gan (postdoctoral researcher)
Presentation by Shuangning Li (postdoctoral researcher)
Presentations by Kelly Zhang (graduate student)
Presentations by Anna Trella (graduate student)
Presentations by Xiang Meng (graduate student)
Presentation by Prasidh Chhabria (undergraduate student)
TR&D2 Lead
Lead PI, Center Director, TR&D1, TR&D2, TR&D3
Co-Investigator, TR&D1, TR&D2
Doctoral Student
Doctoral Student
Research and development by TR&D2 will significantly advance RL methodology for personalizing decision rules; in particular, with regards to online algorithms that personalize interventions for each user by appropriately pooling across multiple users.